Fundamental Valuation
What fundamental measures of business success best indicate the value of individual stocks and the aggregate stock market? How can investors apply these measures to estimate valuations and identify misvaluations? These blog entries address valuation based on accounting fundamentals, including the conventional value premium.
December 6, 2019 - Animal Spirits, Equity Premium, Fundamental Valuation, Momentum Investing
Which equity factors from among those included in the most widely accepted factor models are really important? In their October 2019 paper entitled “Winners from Winners: A Tale of Risk Factors”, Siddhartha Chib, Lingxiao Zhao, Dashan Huang and Guofu Zhou examine what set of equity factors from among the 12 used in four models with wide acceptance best explain behaviors of U.S. stocks. Their starting point is therefore the following market, fundamental and behavioral factors:
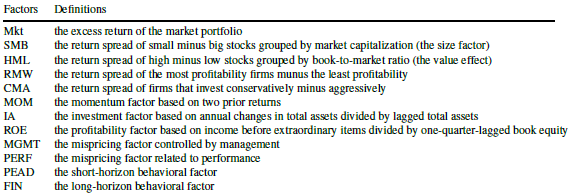
They compare 4,095 subsets (models) of these 12 factors models based on: Bayesian posterior probability; out-of-sample return forecasting performance; gross Sharpe ratios of the optimal mean variance factor portfolio; and, ability to explain various stock return anomalies. Using monthly data for the selected factors during January 1974 through December 2018, with the first 10 (last 12) months reserved for Bayesian prior training (out-of-sample testing), they find that: Keep Reading
December 2, 2019 - Fundamental Valuation
A subscriber requested verification of a fundamental U.S. stock market timing strategy with rebalancing/reallocation of a stocks-bonds portfolio based on Shiller cyclically adjusted price-to-earnings ratio (P/E10 or CAPE) thresholds, as follows:
- If P/E10 > 22, hold 40% stocks and 60% bonds.
- If 14 < P/E10 < 22, hold 60% stocks and 40% bonds.
- If P/E10 < 14, hold 80% stocks and 20% bonds.
The benchmark is an annually rebalanced 60% stocks-40% bonds portfolio (60-40). To assess reasonableness of the P/E10 thresholds chosen, we use P/E10 monthly levels since 1881 and S&P 500 Index monthly returns since 1927. To verify and assess robustness of the specified strategy (P/E10 Timing), we apply it to SPDR S&P 500 (SPY) since inception in 1993 as stocks and Vanguard Long-Term Treasury Investor Shares (VUSTX) as bonds, with monthly rebalancing/reallocation based on P/E10. We consider gross average monthly and annual returns, standard deviations of monthly and annual returns, compound annual growth rate (CAGR), maximum drawdown (MaxDD), and monthly and annual Sharpe ratio as strategy performance metrics. We use monthly and annual average monthly yield on 3-month U.S. Treasury bills (T-bill) to calculate Sharpe ratios. As an additional benchmark, we include a simple technical strategy that is in SPY when prior-month S&P 500 Index is above its 10-month simple moving average and VUSTX when it is below (SPY SMA10). Using the specified inputs, allowing a P/E10 Timing test of nearly 27 years, we find that: Keep Reading
November 15, 2019 - Fundamental Valuation, Momentum Investing
Does including a measure of asset valuation as a qualifier improve the performance of intrinsic (absolute or time series) momentum? In their October 2019 paper entitled “Carry and Time-Series Momentum: A Match Made in Heaven”, Marat Molyboga, Junkai Qian and Chaohua He investigate modification of an intrinsic momentum strategy as applied to futures using the sign of the basis (difference between nearest and next-nearest futures prices) for four asset classes: equity indexes (12 series), fixed income (18 series), currencies (7 series) and commodities (28 series). Their benchmark intrinsic momentum strategy is long (short) assets with positive (negative) returns over the last 12 months, with either: (1) equal allocations to assets, or (2) dynamic allocations that each month target 40% annualized volatility for each contract series. The modified strategy limits long (short) positions to assets with positive (negative) prior-month basis. They account for frictions due to portfolio rebalancing and rolling of contracts using cost estimates from a prior study. They focus on Sharpe ratio to assess strategy performance. Using monthly returns for 65 relatively liquid futures contract series during January 1975 through December 2016, they find that:
Keep Reading
November 11, 2019 - Fundamental Valuation
Does careful accounting for transitory expenses in SEC Form 10-Ks provide a better view of future firm/stock performance than that provided by Generally Accepted Accounting Practices (GAAP) earnings per share (EPS)? In their October 2019 paper entitled “Core Earnings: New Data and Evidence”, Ethan Rouen, Eric So and Charles Wang define Core Earnings, which adds to GAAP 10-K net non-operating expenses related to: (1) acquisitions, (2) currency exchange adjustments, discontinued operations, (4) legal or regulatory events, (5) pension adjustments, (6) restructuring, (7) gains and losses designated “other” by firms and (8) other unclassified gains and losses deemed non-operating. Using a dataset compiled by a combination of human analysts and machine learning that identifies and classifies quantitative disclosures in 10-Ks of Russell 3000 firms, and associated stock prices, during 1998 through 2017, they find that:
Keep Reading
October 9, 2019 - Big Ideas, Fundamental Valuation, Volatility Effects
How does a shift in emphasis from active to passive investing affect the financial market risk landscape? In their September 2019 paper entitled “The Shift From Active to Passive Investing: Potential Risks to Financial Stability?”, Kenechukwu Anadu, Mathias Kruttli, Patrick McCabe, Emilio Osambela and Chaehee Shin analyze how a shift from active to passive investing affects:
- Investment fund redemption liquidity risks.
- Market volatility.
- Asset management industry concentration.
- Co-movement of asset returns and liquidity.
They also assess how effects are likely to evolve if the active-to-passive shift continues. Based on their framework/analysis, they conclude that: Keep Reading
September 25, 2019 - Animal Spirits, Fundamental Valuation
Can investors identify stocks that incorporate news slowly enough to allow exploitation? In their August 2019 paper entitled “Tomorrow’s Fish and Chip Paper? Slowly Incorporated News and the Cross-Section of Stock Returns”, Ran Tao, Chris Brooks and Adrian Bell classify stocks incorporating news quickly (QI) or slowly (SI) into prices and investigate implications for associated future returns. Specifically, they each month:
- Assign a sentiment score to each current-month news article about each stock based on words in the article.
- Double-sort stocks by thirds based first on current-month abnormal (adjusted for size, industry value and industry momentum) returns and then on news sentiment scores, yielding nine groups.
- Classify stocks that are: (a) high return/low sentiment (HRLS) or low return/high sentiment (LRHS) as SI; and, (b) high return/high sentiment (HRHS) or low return/low sentiment (LRLS) as QI.
- Measure average next-month returns of equally-weighted SI and QI portfolios that are, respectively: (a) long LRHS stocks and short HRLS stocks; and, (b) long HRHS stocks and short LRLS stocks.
- Measure average next-month return of an equally weighted portfolio that is long the SI portfolio and short the QI portfolio (Slow-Minus-Quick, SMQ).
They then examine whether limited investor attention or differences in news complexity and informativeness better explain results. Using firm-level news data, firm characteristics and associated stock returns for a broad sample of U.S. common stocks during 1979 through 2016, they find that: Keep Reading
September 24, 2019 - Fundamental Valuation, Value Premium
Why has value investing (long undervalued stocks and short overvalued stocks) performed poorly since 2007? Is it dead, or will it recover? In their August 2019 paper entitled “Explaining the Demise of Value Investing”, Baruch Lev and Anup Srivastava examine the performance of the Fama-French value (HML) factor portfolio, long stocks with high book value-to-market capitalization ratios and short those with low ratios, because it is the most widely used value strategy. They then investigate reasons for its faltering performance. Using value factor returns and accounting data for a broad sample of U.S. stocks during January 1970 through December 2018, they conclude that: Keep Reading
September 18, 2019 - Fundamental Valuation
Do long lags between end of firm quarterly and annual financial reporting periods and issuance of SEC-required financial reports (10-Q and 10-K) indicate internal firm inefficiencies and/or reluctance to disclose adverse performance? In their August 2019 paper entitled “Filing, Fast and Slow: Reporting Lag and Stock Returns”, Karim Bannouh, Derek Geng and Bas Peeters study the impact of reporting lag (number of days between the end of reporting period and filing date of the corresponding report) on future stock returns. They focus on firms with market capitalizations greater than $750 million that have deadlines of 40 days after quarter end for quarterly reports and 60 days after year end for annual reports (accelerated filers). They each month:
- Sort stocks into fifths, or quintiles, based on reporting lag separately for the most recent 10-K and the most recent 10-Q filings.
- Reform a portfolio that is long (short) the equal-weighted quintile with the shortest (longest) lags.
They measure risk-adjusted portfolio performance via monthly gross 1-factor (market), 3-factor (plus size and book-to-market) and 4-factor (plus momentum) alphas. Using 10-K and 10-Q filings from the SEC and monthly characteristics and stock returns for a broad (but groomed) sample of U.S. accelerated filers (roughly 1,500 stocks), and a comparable sample of European stocks, during 2007 through 2018 period, they find that:
Keep Reading
September 13, 2019 - Fundamental Valuation, Technical Trading
Can investors effectively use firm characteristics to screen European stocks? In their August 2019 paper entitled “Predictability and the Cross-Section of Expected Returns: Evidence from the European Stock Market”, Wolfgang Drobetz, Rebekka Haller, Christian Jasperneite and Tizian Otto examine the power of 22 firm characteristics to predict stock returns individually and jointly. They assume market-based characteristics are available immediately and accounting-based characteristics are available four months after firm fiscal year end. For multi-characteristic predictions, they consider 5-characteristic, 8-characteristic and 22-characteristic models. For regression-based forecasts, they use either 10-year rolling or inception-to-date monthly inputs. For economic tests, they form equal-weighted or value-weighted portfolios that are each month long (short) the tenth, or decile, of stocks with the the highest (lowest) expected next-month returns based on 22-characteristic regression outputs. To estimate net performance, they apply one-way trading frictions of 0.57%. Using groomed monthly data for all firms in the STOXX Europe 600 index during January 2003 through December 2018, they find that:
Keep Reading
August 27, 2019 - Animal Spirits, Fundamental Valuation
How does the market react when firms announce adoption of blockchain technology? In the May 2019 draft of their paper entitled “Bitcoin Speculation or Value Creation? Corporate Blockchain Investments and Stock Market Reactions”, Don Autore, Nicholas Clarke and Danling Jiang study stock price reactions to initial public announcements of investments in blockchain technology by listed U.S. firms. Their key metric is buy-and-hold abnormal return (BHAR) relative to each of five benchmarks: (1) portfolios of stocks matched on size and book-to-market (BM); (2) portfolios of stocks matched on market beta; 3) a broad value-weighted market index; (4) iShares Global Financials ETF (IXG); and, (5) iShares Global Tech ETF (IXN). Their announcement event windows is five trading days before initial public announcement of an investment in blockchain technology (-5) to 65 trading days after (65). Using dates of initial public announcements of investments in blockchain technology and contemporaneous daily returns for 207 stocks listed on NYSE and NASDAQ during October 2008 through March 2018, they find that:
Keep Reading